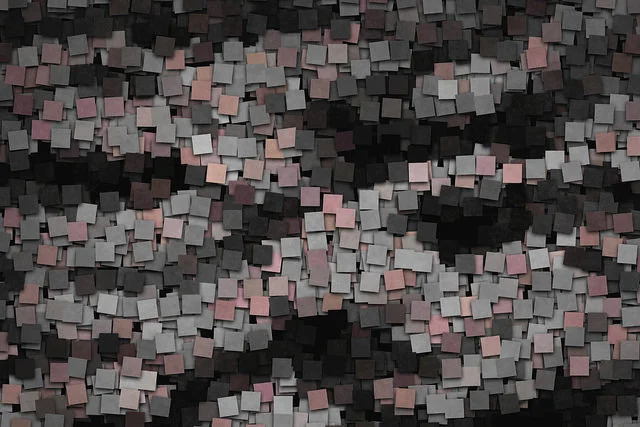
Generatiivinen tekoäly ja tutkimusaineistojen analyysin etiikka
Generatiivinen tekoäly on tutkijalle houkutteleva työkalu. Sen käyttö aineiston analyysissa sisältää kuitenkin tutkimuseettisiä sudenkuoppia: pystyykö tutkija selittämään, miten analyysi on tehty? Kykeneekö arvioija arvioimaan prosessin luotettavuutta? Tiedämmekö, mitä aineistolle tapahtuu, kun sen syöttää tekoälysovellukseen?